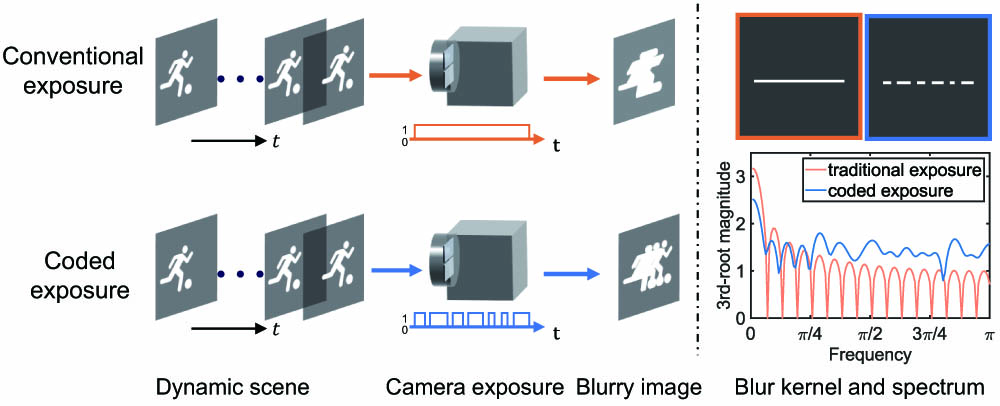
Author Affiliations
Abstract
1 Department of Automation, Tsinghua University, Beijing 100084, China
2 Institute for Brain and Cognitive Sciences, Tsinghua University, Beijing 100084, China
3 Shanghai Artificial Intelligence Laboratory, Shanghai 200030, China
Coded exposure photography is a promising computational imaging technique capable of addressing motion blur much better than using a conventional camera, via tailoring invertible blur kernels. However, existing methods suffer from restrictive assumptions, complicated preprocessing, and inferior performance. To address these issues, we proposed an end-to-end framework to handle general motion blurs with a unified deep neural network, and optimize the shutter’s encoding pattern together with the deblurring processing to achieve high-quality sharp images. The framework incorporates a learnable flutter shutter sequence to capture coded exposure snapshots and a learning-based deblurring network to restore the sharp images from the blurry inputs. By co-optimizing the encoding and the deblurring modules jointly, our approach avoids exhaustively searching for encoding sequences and achieves an optimal overall deblurring performance. Compared with existing coded exposure based motion deblurring methods, the proposed framework eliminates tedious preprocessing steps such as foreground segmentation and blur kernel estimation, and extends coded exposure deblurring to more general blind and nonuniform cases. Both simulation and real-data experiments demonstrate the superior performance and flexibility of the proposed method.
Photonics Research
2023, 11(10): 1678
Yi Zhang 1,2†Yuling Wang 1,2†Mingrui Wang 2,3,4,5Yuduo Guo 2,3,4[ ... ]Qionghai Dai 1,2,4,5,***
Author Affiliations
Abstract
1 Department of Automation, Beijing National Research Center for Information Science and Technology, Tsinghua University, Beijing 100084, China
2 Institute for Brain and Cognitive Sciences, Tsinghua University, Beijing 100084, China
3 Tsinghua Shenzhen International Graduate School, Tsinghua University, Shenzhen 518055, China
4 Beijing Key Laboratory of Multi-dimension & Multi-scale Computational Photography (MMCP), Tsinghua University, Beijing 100084, China
5 IDG/McGovern Institute for Brain Research, Tsinghua University, Beijing, China
6 Hangzhou Zhuoxi Institute of Brain and Intelligence, Hangzhou, 311100, China
Yi Zhang 1,2†Yuling Wang 1,2†Mingrui Wang 2,3,4,5Yuduo Guo 2,3,4[ ... ]Qionghai Dai 1,2,4,5,***
Author Affiliations
Abstract
1 Department of Automation, Beijing National Research Center for Information Science and Technology, Tsinghua University, Beijing 100084, China
2 Institute for Brain and Cognitive Sciences, Tsinghua University, Beijing 100084, China
3 Tsinghua Shenzhen International Graduate School, Tsinghua University, Shenzhen 518055, China
4 Beijing Key Laboratory of Multi-dimension & Multi-scale Computational Photography (MMCP), Tsinghua University, Beijing 100084, China
5 IDG/McGovern Institute for Brain Research, Tsinghua University, Beijing, China
6 Hangzhou Zhuoxi Institute of Brain and Intelligence, Hangzhou, 311100, China
High-speed visualization of three-dimensional (3D) processes across a large field of view with cellular resolution is essential for understanding living systems. Light-field microscopy (LFM) has emerged as a powerful tool for fast volumetric imaging. However, one inherent limitation of LFM is that the achievable lateral resolution degrades rapidly with the increase of the distance from the focal plane, which hinders the applications in observing thick samples. Here, we propose Spherical-Aberration-assisted scanning LFM (SAsLFM), a hardware-modification-free method that modulates the phase-space point-spread-functions (PSFs) to extend the effective high-resolution range along the z-axis by ~ 3 times. By transferring the foci to different depths, we take full advantage of the redundant light-field data to preserve finer details over an extended depth range and reduce artifacts near the original focal plane. Experiments on a USAF-resolution chart and zebrafish vasculatures were conducted to verify the effectiveness of the method. We further investigated the capability of SAsLFM in dynamic samples by imaging large-scale calcium transients in the mouse brain, tracking freely-moving jellyfish, and recording the development of Drosophila embryos. In addition, combined with deep-learning approaches, we accelerated the three-dimensional reconstruction of SAsLFM by three orders of magnitude. Our method is compatible with various phase-space imaging techniques without increasing system complexity and can facilitate high-speed large-scale volumetric imaging in thick samples.
Author Affiliations
Abstract
1 Department of Automation, Tsinghua University, 100084 Beijing, China
2 Research Center for Industries of the Future and School of Engineering, Westlake University, 310024 Hangzhou, China
3 Key Laboratory of 3D Micro/Nano Fabrication and Characterization of Zhejiang Province, Westlake University, 310024 Hangzhou, China
4 Computer Network Information Center, Chinese Academy of Sciences, 100190 Beijing, China
5 University of Chinese Academy of Sciences, 100049, Beijing, China
6 Wyant College of Optical Sciences, University of Arizona, AZ 85721 Tucson, USA
High-throughput imaging is highly desirable in intelligent analysis of computer vision tasks. In conventional design, throughput is limited by the separation between physical image capture and digital post processing. Computational imaging increases throughput by mixing analog and digital processing through the image capture pipeline. Yet, recent advances of computational imaging focus on the “compressive sampling”, this precludes the wide applications in practical tasks. This paper presents a systematic analysis of the next step for computational imaging built on snapshot compressive imaging (SCI) and semantic computer vision (SCV) tasks, which have independently emerged over the past decade as basic computational imaging platforms. SCI is a physical layer process that maximizes information capacity per sample while minimizing system size, power and cost. SCV is an abstraction layer process that analyzes image data as objects and features, rather than simple pixel maps. In current practice, SCI and SCV are independent and sequential. This concatenated pipeline results in the following problems: i) a large amount of resources are spent on task-irrelevant computation and transmission, ii) the sampling and design efficiency of SCI is attenuated, and iii) the final performance of SCV is limited by the reconstruction errors of SCI. Bearing these concerns in mind, this paper takes one step further aiming to bridge the gap between SCI and SCV to take full advantage of both approaches. After reviewing the current status of SCI, we propose a novel joint framework by conducting SCV on raw measurements captured by SCI to select the region of interest, and then perform reconstruction on these regions to speed up processing time. We use our recently built SCI prototype to verify the framework. Preliminary results are presented and the prospects for a joint SCI and SCV regime are discussed. By conducting computer vision tasks in the compressed domain, we envision that a new era of snapshot compressive imaging with limited end-to-end bandwidth is coming.

Author Affiliations
Abstract
1 School of Electronic Science and Engineering, Nanjing Universityhttps://ror.org/01rxvg760, Nanjing 210023, China
2 Department of Automation, Tsinghua University, Beijing 100084, China
3 Institute for Brain and Cognitive Sciences, Tsinghua University, Beijing 100084, China
4 Beijing Institute of Collaborative Innovation, Beijing 100094, China
5 Department of Biomedical Engineering, University of Connecticut, Storrs, Connecticut 06269, USA
6 e-mail: qhdai@mail.tsinghua.edu.cn
7 e-mail: caoxun@nju.edu.cn
Micro-endoscopes are widely used for detecting and visualizing hard-to-reach areas of the human body and for in vivo observation of animals. A micro-endoscope that can realize 3D imaging at the camera framerate could benefit various clinical and biological applications. In this work, we report the development of a compact light-field micro-endoscope (LFME) that can obtain snapshot 3D fluorescence imaging, by jointly using a single-mode fiber bundle and a small-size light-field configuration. To demonstrate the real imaging performance of our method, we put a resolution chart in different positions and capture the z-stack images successively for reconstruction, achieving 333-μm-diameter field of view, 24 μm optimal depth of field, and up to 3.91 μm spatial resolution near the focal plane. We also test our method on a human skin tissue section and HeLa cells. Our LFME prototype provides epi-fluorescence imaging ability with a relatively small (2-mm-diameter) imaging probe, making it suitable for in vivo detection of brain activity and gastrointestinal diseases of animals.
Photonics Research
2022, 10(9): 2247
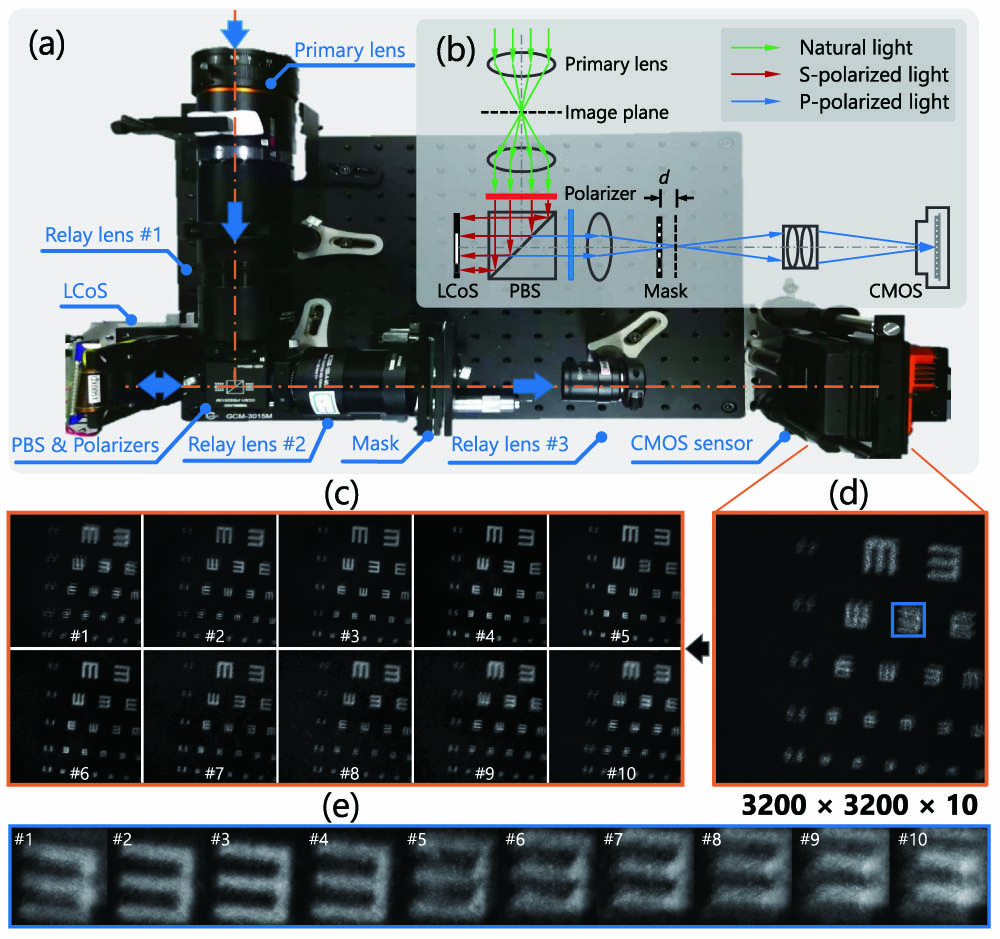
Author Affiliations
Abstract
1 Department of Automation, Tsinghua University, Beijing 100084, China
2 Institute for Brain and Cognitive Science, Tsinghua University, Beijing 100084, China
3 Computer Science and Artificial Intelligence Laboratory, Massachusetts Institute of Technology, Cambridge, Massachusetts 02139, USA
4 Westlake University, Hangzhou 310024, China
5 Beijing National Research Center for Information Science and Technology, Tsinghua University, Beijing 100084, China
6 e-mail: xyuan@westlake.edu.cn
High-resolution images are widely used in our everyday life; however, high-speed video capture is more challenging due to the low frame rate of cameras working at the high-resolution mode. The main bottleneck lies in the low throughput of existing imaging systems. Toward this end, snapshot compressive imaging (SCI) was proposed as a promising solution to improve the throughput of imaging systems by compressive sampling and computational reconstruction. During acquisition, multiple high-speed images are encoded and collapsed to a single measurement. Then, algorithms are employed to retrieve the video frames from the coded snapshot. Recently developed plug-and-play algorithms made the SCI reconstruction possible in large-scale problems. However, the lack of high-resolution encoding systems still precludes SCI’s wide application. Thus, in this paper, we build, to the best of our knowledge, a novel hybrid coded aperture snapshot compressive imaging (HCA-SCI) system by incorporating a dynamic liquid crystal on silicon and a high-resolution lithography mask. We further implement a PnP reconstruction algorithm with cascaded denoisers for high-quality reconstruction. Based on the proposed HCA-SCI system and algorithm, we obtain a 10-mega-pixel SCI system to capture high-speed scenes, leading to a high throughput of 4.6 × 109 voxels per second. Both simulation and real-data experiments verify the feasibility and performance of our proposed HCA-SCI scheme.
Photonics Research
2021, 9(11): 11002277
Author Affiliations
Abstract
1 Department of Automation, Tsinghua University, Beijing 100084, China
2 Institute for Brain and Cognitive Science, Tsinghua University, Beijing 100084, China
3 Tsinghua Shenzhen International Graduate School, Tsinghua University, Shenzhen 518055, China
4 Beijing Innovation Center for Future Chip, Tsinghua University, Beijing 100084, China
5 Institute of Microelectronics, Tsinghua University, Beijing 100084, China
6 Beijing National Research Center for Information Science and Technology, Tsinghua University, Beijing 100084, China
7 e-mail: lin-x@tsinghua.edu.cn
8 e-mail: qhdai@tsinghua.edu.cn
This publisher’s note corrects the authors’ affiliations in
Photon. Res.8, 940 (2020).PRHEIZ2327-912510.1364/PRJ.389553Photonics Research
2020, 8(8): 08001323

Author Affiliations
Abstract
1 Department of Automation, Tsinghua University, Beijing 100084, China
2 Institute for Brain and Cognitive Science, Tsinghua University, Beijing 100084, China
3 Tsinghua Shenzhen International Graduate School, Tsinghua University, Shenzhen 518055, China
4 Beijing Innovation Center for Future Chip, Tsinghua University, Beijing 100084, China
5 Institute of Microelectronics, Tsinghua University, Beijing 100084, China
6 Beijing National Research Center for Information Science and Technology, Tsinghua University, Beijing 100084, China
7 e-mail: lin-x@tsinghua.edu.cn
8 e-mail: qhdai@tsinghua.edu.cn
Training an artificial neural network with backpropagation algorithms to perform advanced machine learning tasks requires an extensive computational process. This paper proposes to implement the backpropagation algorithm optically for in situ training of both linear and nonlinear diffractive optical neural networks, which enables the acceleration of training speed and improvement in energy efficiency on core computing modules. We demonstrate that the gradient of a loss function with respect to the weights of diffractive layers can be accurately calculated by measuring the forward and backward propagated optical fields based on light reciprocity and phase conjunction principles. The diffractive modulation weights are updated by programming a high-speed spatial light modulator to minimize the error between prediction and target output and perform inference tasks at the speed of light. We numerically validate the effectiveness of our approach on simulated networks for various applications. The proposed in situ optical learning architecture achieves accuracy comparable to in silico training with an electronic computer on the tasks of object classification and matrix-vector multiplication, which further allows the diffractive optical neural network to adapt to system imperfections. Also, the self-adaptive property of our approach facilitates the novel application of the network for all-optical imaging through scattering media. The proposed approach paves the way for robust implementation of large-scale diffractive neural networks to perform distinctive tasks all-optically.
Photonics Research
2020, 8(6): 06000940
光场为三维世界中光线集合的完备表示。通过记录更高维度的光线数据,光场能够准确感知周围复杂多变的动态环境,支撑智能系统对环境的理解与决策。计算光场成像技术围绕光场及全光函数表示,旨在结合计算、数字传感器、光学系统和智能光照等技术,以及硬件设计、软件计算能力,突破经典成像模型和数字相机的局限性,建立光在空域、视角、光谱和时域等多个维度的关系,实现耦合感知、解耦重建与智能处理,具备面向大范围动态场景的多维多尺度成像能力。光场成像技术正逐渐被应用于生命科学、工业探测、****、无人系统和虚拟现实/增强现实等领域,具有重要的学术研究价值和广阔的产业应用前景。然而,伴随着高维数据的离散化采样,光场成像面临空间分辨率与视角分辨率的维度权衡挑战,如何对稀疏化的采样数据进行光场重建成为计算光场成像及其应用的基础难题。与此同时,受制于光场信号的高维数据感知量,光场处理面临有效数据感知与计算高效性的矛盾。如何用光场这一高维信息采集手段,取代传统二维成像视觉感知方法,并结合智能信息处理技术实现智能化高效感知,是实现光场成像技术产业化应用的巨大挑战。对过去20年来计算光场成像装置与算法的研究进行概述和讨论,内容涵盖光场表示和理论、光场信号采集、空间与视角维度重建等。
成像系统 计算摄像 光场成像 十亿像素 阵列相机
1 清华大学 自动化系, 北京 100084
2 西安高科技研究所, 陕西 西安 710025
单像素成像旨在通过单个感光元件记录目标场景信息。由于其高灵敏度、宽谱段响应等良好的特性, 单像素成像是近年来的研究热点。通过对高维光信号的编码采集与解码重构, 单像素成像能够满足丰富的成像需求。介绍了单像素成像的研究背景, 简述了其成像原理及重构算法, 从光信息编码与解码角度系统回顾了单像素成像的研究现状和前沿技术。此外, 还讨论了单像素成像技术中存在的问题, 以及未来可能的研究方向与应用。
单像素成像 光信息 编码采集 解码重构 single-pixel imaging optical information coded acquisition decoded reconstruction 红外与激光工程
2019, 48(6): 0603004